Past Project
Monitoring water quality and modelling ecosystem dynamics in a freshwater environment serve as useful decision making tools by assessing the ecological status and informing current and future outcomes of environmental management actions. However, uncertainties occur from various sources in the process of monitoring and modelling, which makes the decision making difficult. This research aims to quantify and investigate characteristics of such uncertainties and study how to effectively inform decision makers about the uncertainties.
Low measurement frequency and consequent sampling bias hinders from accurately and precisely assessing the status of freshwater ecosystems. Nutrient transfer models and statistical inference models bear uncertainties of greater extent because the uncertainties in the data used in the model propagate (aleatoric uncertainty) and assumptions of their structures are subject and can be incorrect (epistemic uncertainty).
In this project, 1) statistical models for inferring physico-chemical status of river are tested, 2) temporal evolution of nitrogen transfer after an ecological disturbance is inferred using a parsimonious catchment model, and 3) types of phosphorus transfer are identified using an empirical concentration-discharge hysteresis model. Aleatoric and epistemic uncertainties in the hydrochemical monitoring and modelling are quantified using Bayesian statistics or high-frequency data. Decision making uncer the uncertainties are discussed.
This project is funded by the Humboldt University of Berlin.
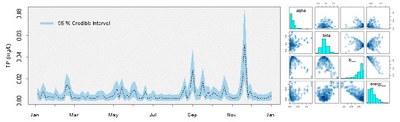
Output
Arce, M. I., D. von Schiller, M. M. Bengtsson, C. Hinze, H. Jung, R. Alves, T. Urich, and G. Singer (2018), Drying and Rainfall Shape the Structure and Functioning of Nitrifying Microbial Communities in Riverbed Sediments, Front. Microbiol, 9, 2797. https://doi.org/10.3389/fmicb.2018.02794
Jung, H., C. Senf, P. Jordan, and T. Krueger (2020), Benchmarking inference methods for water quality monitoring and status classification, Environmental monitoring and assessment, 192(4), 1-17. https://doi.org/10.1007/s10661-020-8223-4
Jung, H., C. Senf, B. Beudert, and T. Krueger (2021), Bayesian hierarchical modeling of nitrate concentration in a forest stream affected by large‐scale forest dieback, Water Resources Research, 57(2), e2020WR027264. https://doi.org/10.1029/2020WR027264